Goals
Inference models vary in complexity and required customization. For example, a simple descriptive study may only require correlations (e.g. Pearson correlation) or the use of a traditional nonparametric model (e.g. multivariate regression). Or perhaps the study is an experiment attempting to understand a causal relationship that requires the use of a more customized graphical model (e.g. Markov Random Fields). In all cases, we need to assess the soundness of the selected model or determine if an appropriate model (algorithm) does not exist and a new one needs to be developed. In some cases, we also need to train the model using labeled data and tune the model parameters. This is a process that is well understood in computer science. Unfortunately, social media data have new forms of uncertainty – including non-random noise, partial information, and misinformation – that are not well understood. While there is a growing literature about the increasing impact of misinformation and the importance of finding ways to correct it, we still do not understand how randomly these new forms of uncertainty are distributed or their impact on the construction of different models, particularly longitudinal ones. There are also biases that are specific to different social media portals, e.g. times of day people post, types of posts that are common, etc. These need to be considered during model construction.
Meeting Summary
The meeting took place online and had 21 attendees, including nine guests from outside the project team who spent a day and a half discussing, presenting, and writing about model construction issues. The team is now drafting a white paper about the modeling challenges associated with research involving social media data. We are also updating our interactive glossary of terms used differently across different disciplines, and growing our new Google group discussing social media research.
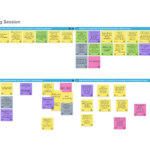
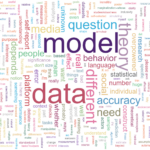
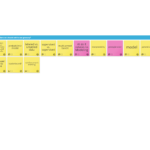
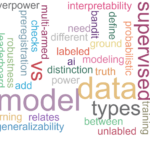