Goals
To use data from social media sources, or any organic data, in an effective way, social scientists must generate measures or variables (e.g., topic or sentiment) to describe and quantify the information contained in the data. To create these measures, social scientists must use computer science techniques because traditional manual coding of text data is simply not feasible with “big data”. Feature engineering focuses on how we construct these different variables from text, while data curation focuses on the organization, integration, and preprocessing of data collected from various sources. For example, we can use opinion mining to determine a stance or position of a user about a topic given a specific “tweet” or “post”, e.g. a user’s opinion on vaccination. However, the machine learning algorithms used by computer scientists have inconsistent standards for establishing the validity or reliability of variables generated from social media data. To use these variables in social science research in a way that would be acceptable to a wider scholarly community, the validity and reliability of the constructed data needs to be assessed through some sort of “ground truthing” process. This meeting will focus on issues faced by social, computer and data scientists in thinking about these and other measurement issues.
Meeting Summary
The meeting took place online and had 22 attendees, including ten guests from outside the project team who spent a day and a half discussing, presenting, and writing about measurement issues. The team wrote a white paper about the measurement challenges associated with research involving social media data. We are also updating our interactive glossary of terms used differently across different disciplines, and growing our new Google group discussing social media research.
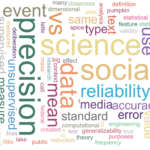
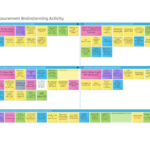
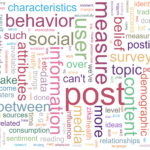
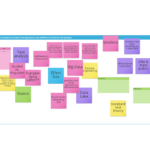